Meet our Bioinformatics team: Genomics Data Scientist
By Vasavi Sundaram on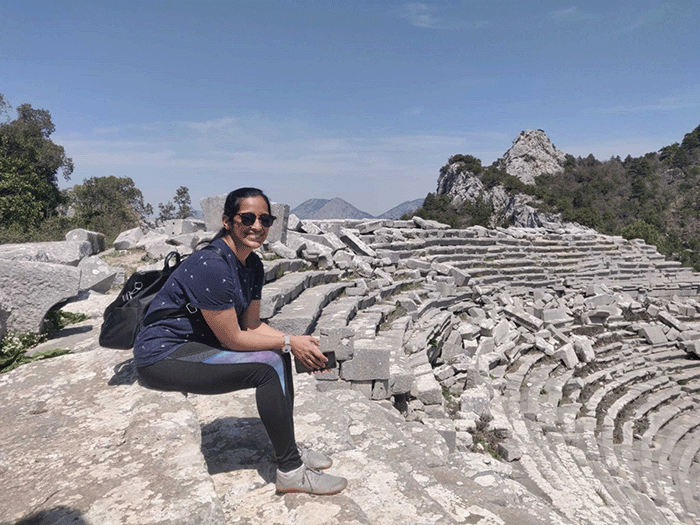
Vasavi Sundaram, Genomics Data Scientist
The Genome Analysis team at Genomics England are working to develop cutting-edge genome analysis approaches for diverse clinical applications. They aim to enable world-leading personalised healthcare that is accessible for everyone. We spoke to our Genomics Data Scientist Vasavi Sundaram about her current role and how genomics training has helped to shape her technical and scientific skills.
How would you describe your role and what does your typical working day look like?
My work as a Genomics Data Scientist can be split into three main activities: analysis, meetings, and learning. Most of the work is ‘discovery’ in nature, where we gather evidence to improve the variant prioritisation in our pipeline and report it for clinicians. Along with others in the team, I work on benchmarking the performance of somatic variant calling in our pipeline.
Out of the three main activities, analyses take up most of my time. In a typical day I get a few hours ‘meeting-free’ so I can write or run code, generate plots and summary statistics, and document results for the team. We aim to write code that can be reused wherever possible and is fairly simple to reproduce. At Genomics England, most teams are multi-disciplinary and work in a hybrid manner. This means that meetings are integral for us to address barriers, give feedback, and ensure that team deliverables are completed on time. We also have weekly, fortnightly, or monthly meetings with internal and external collaborators to receive feedback throughout the process of developing pipeline improvements.
In addition to these activities, Genomics England pays strong attention to individual development. Towards this effort, we have weekly learning time, biweekly journal clubs or skill-share sessions, and research seminars where we share and advance our knowledge in genomic research.
Tell us about your educational background
98% of the human genome is made up of non-coding DNA sequences. During my academic training, I worked in epigenomics to try and understand the role of this non-coding DNA and how it regulates gene expression across different species and tissues. Malfunctions in gene expression regulation are often associated with tumour development; I chose to investigate this as the main focus of my postdoctoral research. Following this, I wanted to further my research experience with more application of using sequencing technology to enhance patient clinical outcomes.
I looked for opportunities to work on applying advances in genomics to the diagnosis and treatment of cancer. Considering the reduced cost of whole-genome sequencing datasets and the wide availability of algorithms to analyse these datasets, I was keen to help bring advances to the patient. At Genomics England, I get to apply my genomics training to extract insights from whole-genome sequencing of tumour samples and use this to identify clinically actionable variants for cancer patients. I also get to work on diverse aspects of cancer genomics in a multi-disciplinary team. This enables the return of clinically relevant genomic information to clinicians, thereby improving diagnostic accuracy. Academia and companies differ in many ways, but doing research is fundamentally the same!
What skills do you think are most important to be successful in your role?
Along with colleagues and others in the field, I used next-generation sequencing technologies to profile the ‘biological function’ of the non-coding genome throughout my academic training. Functional interpretation of the genome involves integrating large and diverse datasets to extract signals by using various algorithms and statistical tests. The skills I acquired throughout this training can be summarised into three main aspects: technical, scientific, and communication.
Technically, data analysis includes a lot of coding and synthesising datasets into meaningful features in the genomic regions of interest. Coding in python/shell and plotting in R were my choices for data analysis. Often, analyses include using published software and applying statistical tests to the biological data in hand; a scientific understanding of the consequences of these statistical tests is very important. Furthermore, documentation is also central to research and technical work to ensure that research can be reproduced. Finally, research is dependent on a community that collaborates. Communicating and presenting your research to the wider community is beneficial for getting feedback and sharing knowledge.
If you had to choose a career other than genomics data science, what would it be?
Probably something along the lines of architecture, sports science, or molecular gastronomy.