Understanding genetic links to disease by mapping variants to genes
By Elena Bernabeu and Chris Odhams onThe Bioinformatics Research Services' Bioconsulting team (BRSC) collaborates with academic and commercial partners to help unravel the genetic underpinnings of different diseases. Part of this research involves the mapping of variants to genes to better understand the mechanisms that link genes with disease. In this blog, Elena Bernabeu (Bioinfomatician within BRSC) introduces the context surrounding variant-to-gene mapping, sharing the approach and information that her team uses to carry out the task.
Genome-wide association studies (GWAS): what next?
A genome-wide association study (GWAS) is a vital method used to capture how a common genetic variant might be associated with disease. GWASs query variants across the entire genome, and use large case-control cohorts to evaluate which variants are associated with an increased or decreased risk for a given disease. These studies have found great success, and have identified hundreds of thousands of variants all over the genome that are associated with a plethora of diseases.
However, this is only the beginning. Often, an association found between a variant and a disease does not directly tell us what is happening biologically. Additionally, because of a phenomenon termed linkage disequilibrium (LD), a variant might be linked/correlated with other nearby variants, and it can be hard to determine which variant is truly driving the disease association.
Non-coding associations: what’s going on?
Put simply, the human genome is made up of protein-coding genes and the non-coding space between them. The majority of the human protein-coding genome has been characterised, meaning we know which proteins the genes in our DNA encode, and for a large portion of them, we also know their function. On the other hand, characterisation and understanding of the non-coding portion of the genome has been more challenging.
One reason the biological interpretation of GWAS signals has been difficult, is that the majority of disease-associated variants fall within the non-coding genome. When a variant falls within a coding sequence, researchers can predict its effect on protein structure and function. But when a genetic variant falls within this "unknown" non-coding DNA, interpretation is more challenging.
Genetic variants in non-coding regions are thought to mediate disease risk through gene regulation – for example, by altering gene expression. Pinpointing which gene a variant is mediating could thus help understand genetic links to disease, increase our understanding of disease pathogenesis, and potentially highlight novel drug targets.
For these reasons, variant to gene mapping efforts have been extensively researched in the genetics field in recent years, to help us bridge the gap between GWAS and disease.
Variant to gene mapping: finding the clues
Let’s assume we have performed a GWAS on a disease and found a novel associated variant. We assume the causal gene that is mediating our variant’s effect is nearby in the genome, as this is typically the case. As such, we define a window around our variant and find all genes within it. These make up our candidate causal genes.
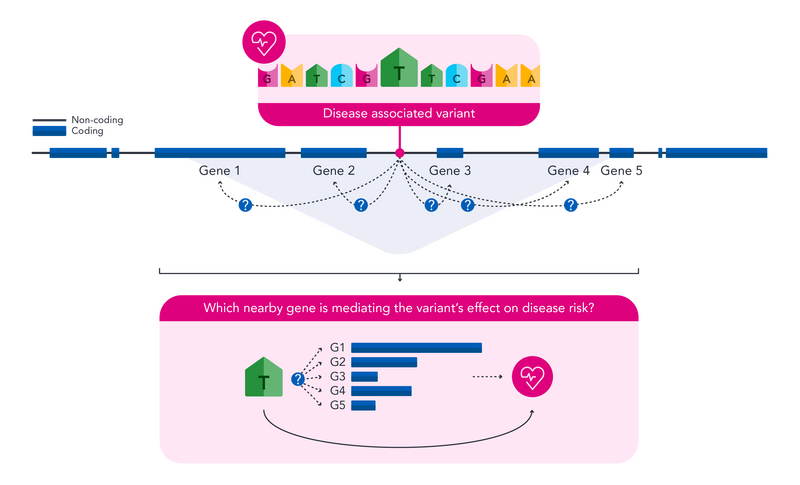
Variant to gene mapping involves the accumulation of multiple sources of information that can be used to identify potential disease-mediating/causal genes, as well as better understand variant-disease links. We divide up mapping "clues" into those that can be found at the variant level, as well as at the gene level.
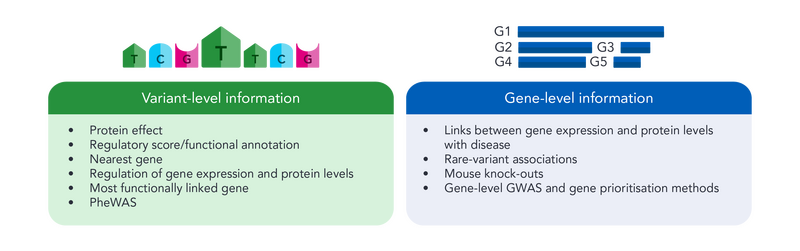
Here, we touch upon multiple information sources that we weigh when mapping variants to genes within the Bioconsulting team.
Variant level information
Protein effect
The associated variant or highly correlated variants in LD may fall within the coding sequence of a gene. Some coding variants can have more extreme consequences on protein structure and function than others, and some might not have any effect at all. Several scores can be used to evaluate the severity of a variant, including those by VEP (Variant Effect Predictor) or CADD (Combined Annotation Dependent Depletion). If a variant within the coding sequence is predicted to have a large effect on the protein, for example by impeding it from doing its normal function, the gene affected is a good candidate to be the causal gene for the disease studied.
Regulatory score/functional annotation
Some non-coding regions of the genome are known to be regulatory elements. For example, they might bind regulatory proteins that then lead to over or under expression of a gene. As such, the associated variant or highly correlated variants in LD in these regions might affect the levels of a protein if binding is disrupted. This may lead to changes in phenotype/disease presentation. We can assess if our variant falls within these regulatory regions using resources like RegulomeDB, which returns a score after assessing the evidence a variant has for regulatory potential.
Nearest gene
Variants can regulate both nearby and faraway genes. However, evidence suggests that the nearest gene is most often the target of a non-coding regulatory variant. As such, distance from the variant to genes can serve as a clue when finding our causal gene.
Regulation of gene expression and protein levels
GWASs query the association between all variants in the genome and the manifestation of a given disease. This same method can be applied to find variants that regulate gene expression or protein levels in eQTL and pQTL studies (expression/protein quantitative trait loci, respectively).
eQTL and pQTL studies can help understand what non-coding variants do. If a GWAS variant is found to regulate expression/protein levels of a gene in a tissue relevant to the disease under study, this could indicate that the gene plays a causal role in the disease. Publicly available eQTL and pQTL study summary statistics, obtained in large studies such as the UK Biobank or GTEx, are available via platforms like Open Targets, which gathers results from multiple studies and can be easily queried.
Most functionally linked gene
Variant-level information can be used together to identify the most likely candidate gene. Open Targets offers users the results of their bespoke Variant to Gene (V2G) pipeline, which uses QTL, distance to a gene, and regulatory data to find the most functionally linked gene to a given variant. This is a powerful tool that can aid in the finding of the causal gene.
PheWAS
The same way we look for associations between all variants and a single phenotype in a GWAS, we can query all phenotypes to assess associations with a single variant. This framework is called a phenome-wide association study (PheWAS), and it can help identify diseases that a variant has been linked with. This can further inform on the function of a variant, for example, by informing on associations with potential intermediary phenotypes, or highlighting shared mechanisms amongst different diseases.
Additionally, if a variant/gene was found to be a viable drug target, these analyses could highlight repurposing opportunities. A PheWAS can be carried out directly by the researcher, or GWAS resources can be queried. Open Targets offers the possibility of querying multiple studies to identify traits associated with a given variant.
Links between gene expression and protein levels with disease
Many studies have investigated the differences in either gene expression or protein levels between diseased and non-diseased individuals. Whilst highly informative, these studies are relatively small and do not directly assess the genetic effects underlying the observed differences. To determine this, the transcription-wide association study (TWAS) was developed. This uses reference panels to predict gene expression in individuals from large populations and then uses GWAS results to assess whether this predicted measurement is associated with a disease.
Parallel methods such as Mendelian Randomisation (MR) and colocalisation, can further establish if there is a causal link between gene expression/protein levels and disease, and whether there is a shared causal variant between each trait. If a gene near our variant presents a significant association with a disease using these methods, it provides evidence for it to be the underlying causal gene.
Rare variant associations
Rare variants which are not included in GWASs may present larger effects on disease than common variants. As such, their enrichment in a gene might indicate disruption in its function. For this reason, querying whether rare variant links have been reported with diseases relevant to that studied in our GWAS might point to genes that could be acting as genetic mediators of disease. One potential source of information for rare variant associations is the AstraZeneca PheWAS Portal.
Similarly, links between a gene and a relevant Mendelian disease, driven by rare variants, might also point to the most likely causal gene. This can be checked with resources like ORPHANET.
Mouse knock-outs
Mouse gene knock-outs can help identify gene function. If the considered gene has an equivalent counterpart (termed ortholog) in mouse models, this gene can be ‘knocked-out’ (meaning inactivated) in a controlled experiment, and the consequences on the organism studied. Indeed, if a knock-out consequence for the studied gene has been reported that is relevant to the disease in question, this could be a clue linking the gene to the disease of interest. A potential source for this information is the Mouse Phenome Database.
Gene-level GWAS and gene prioritisation methods
Methods exist to aggregate GWAS results, which are at variant-level, to obtain gene-level association statistics. This can serve as an additional layer of information to help pinpoint the causal gene.
The method MAGMA can be layered with additional information making use of methods like PoPS. PopS builds on gene-level GWAS summary statistics to return a gene prioritisation score for all genes, using information from gene expression experiments, protein-protein interaction networks, and pathway membership.
Bringing the evidence together
Once we’ve collected our evidence, we can ask the following questions for all candidate genes:
- Does the gene contain a protein altering variant that’s highly correlated with/is our lead variant?
- Is the gene the one nearest to our lead variant?
- Is the gene’s expression or protein levels regulated by our lead variant (eQTL/pQTL)?
- Has the gene been causally linked to disease (TWAS/MR)?
- Has the gene been linked to disease via GWAS gene-agglomerating methods (MAGMA)?
- Does the gene present differential expression/protein levels in cases/controls for this disease?
- Has a rare-variant association been reported for this gene in a relevant disease?
- Is this gene linked to a Mendelian disease that is related to the disease under study?
- Is the gene a human ortholog of a mouse-knockout gene with a relevant phenotype?
- What is the gene’s prioritisation score from gene prioritisation methods like PoPS?
- Is this the gene that’s been most functionally linked to the lead variant by Open Target’s V2G pipeline?
All this information can be used in different ways. For example, we could bring forward the most likely causal gene which met the most of the above criteria, though other, more complex, methods exist. Ultimately, we will identify a single or multiple potential causal genes for each associated variant to bring forward for additional downstream analyses.
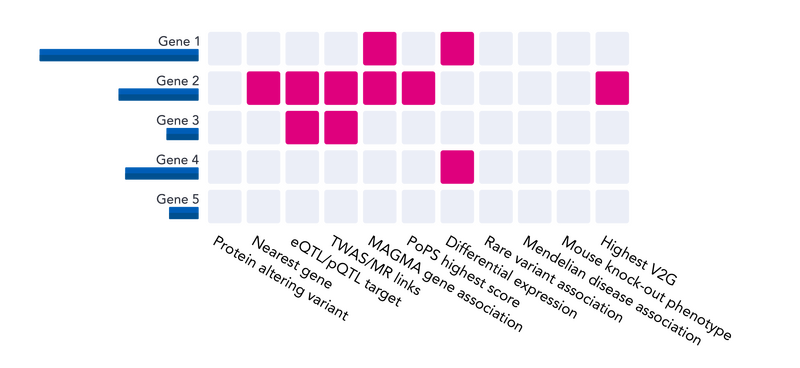
Candidate genes as potential drug targets
Our ultimate goal with these efforts is to further understand disease mechanisms and discover novel drug targets.
The candidate genes that are found can serve as starting points for the discovery of the latter. However, not every gene/protein is equally targetable, and some might already be the target of existing drugs. This can be evaluated using resources like chEMBL or the Drug Gene Interaction Database. Protein-protein interactions can also be considered to assess potential side-effects and/or to find alternative drug targets, using resources such as STRINGdb.
Further information on disease mechanism can be found by expanding our view beyond the gene level and into the pathway and network level. This brings us closer to understanding the complex relationships amongst all genetic associations found in a GWAS for a given disease, and disease aetiology in general.
Finally, before any findings can be brought downstream for further investigation, targets should be validated in vivo, for example making use of CRISPR technology and mouse models.
Ultimately, variant to gene mapping is just a (very large and key) piece of the puzzle that is understanding the underlying genetics of disease. Further improvements can be made in this field, and with the increased populating of databases and the generation of single-cell multi-omics datasets in ever-larger and more diverse populations, the future is bright.
Read other bioinformatics blogs on the Genomics England website.