New review on statistical and machine learning tools to advance equity in genomic research
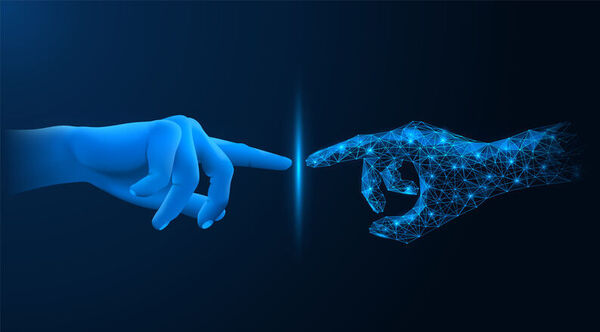
A review funded as part of Genomics England’s Diverse Data initiative and led by UCL researchers has outlined how the analytical methods used to process and interpret genomic data play a critical role in promoting health equity.
The review, published in Nature Reviews Genetics, also discusses how current statistical and machine learning tools can either worsen or help correct biases and calls for more equitable methods to ensure genomic research benefits all populations fairly.
The Diverse Data initiative aims to reduce health inequalities and improve patient outcomes in genomic medicine for minoritised communities. A key issue within genomics is the current underrepresentation of certain groups in existing databases, with the analytical methods used to process, analyse and interpret genomic data an often-overlooked source of inequity. Improving this can lead to a range of benefits including a better understanding of genes linked to illness as well as new treatments that work for a more diverse group of people.
One focus area of Diverse Data is emerging technologies and methods – specifically exploring new approaches and technologies and how they can further our understanding of human genetic variation.
The review undertaken by the research team examines how bias can enter each step involved in genomic data analysis, from research design and data acquisition to data preparation, model development and evaluation. The growing appreciation of the impact of existing biases has seen the development of new statistical techniques to understand, quantify and correct for imperfect data and models.
For instance, given the current lack of diversity in genomic datasets, methods to boost power for statistical inference or prediction in under-represented groups can provide large benefits in terms of equity. The paper highlights three strategies to boost power and specific methodological techniques: including more individuals, including more traits, and leveraging non-genetic data. The paper also explores how statistical methods can reduce bias, assess genetic variation, and identify disparities in existing analysis pipelines.
The review also lays out further issues related to categorisation, genomic references, data sharing and understanding the role of social and environmental effects.
“This research shows just how important the analytical methods used to process and interpret genomic data can be in ensuring everyone can access genomics’ benefits equally.
“The Diverse Data programme commissioned this project because we recognise that equity in health research needs to improve. The findings shine a light on a source of inequalities within genomics that is frequently disregarded, whilst also highlighting how the research community is coming together to develop innovative new methods to tackle these challenges. We hope others in the field will find the recommendations the project has put forward helpful for their own analyses.”
Sam Tallman
Co-author of the paper from Genomics England