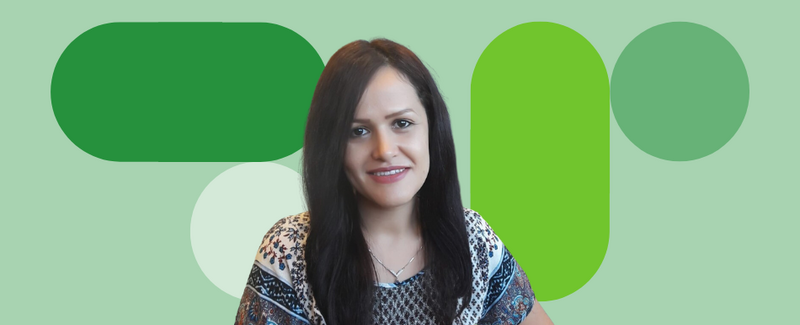
Machine learning expert Golestan Karami shares how data from the National Genomic Research Library is helping her improve predictions of patient outcome by studying the imaging features and genetic features of cancer.
What I am currently working on
For many years I worked in hospitals as a medical physicist. I used to work in the radiology and radiotherapy department, enhancing my knowledge of cancer imaging and treatment.
I then completed a PhD in machine learning, and became interested in developing machine learning models that use data from an imagining technique called magnetic resonance imaging (MRI).
I am currently researching whether machine learning models that use multiple types of data could enhance our understanding of tumour behaviour. Specifically, I am looking at glioblastoma, a cancer that originates in the brain and is typically very aggressive.
Understanding tumour behaviour could improve numerous aspects of medical diagnosis, treatment, and patient care.
For example, we could better predict how well a patient is likely to respond to treatment, and what the best course of action might be.
The challenge is identifying which treatments fit which individuals
Currently, it is unclear who will respond best to what treatment. We can’t just look at patients and know how they will react.
To address this challenge, we are exploring the integration of multiomic data – which includes genomics, imaging and clinical data – to investigate potential associations between genetic alterations, tumour characteristics and patient outcome.
Using machine learning algorithms, we aim to identify any correlations or patterns that could shed light on the relationship between specific genetic changes and tumour phenotype.
This approach, known as radiogenomics, has potential to provide valuable insights into molecular mechanisms behind gliomas, and aid the development of personalised diagnostic and treatment strategies.
More than meets the eye: using machine learning in cancer research
If multiple radiologists examine a series of MRI images, they may not always arrive at the same conclusion. The human eye cannot detect and interpret all the information present in MRI images.
Genomic data presents a similar challenge. It is vast and complex, so manually analysing or interpreting this data can be limited by human biases. This is where machine learning is valuable.
The idea is that if images were to be processed with statistical analysis as opposed to just the human eye, we could reach one singular conclusion as opposed to different ones from different people.
If we can figure this process out for a proper dataset, then we should be able to apply it to other datasets as well.
The value of the National Genomic Research Library
The National Genomic Research Library has been so helpful and important in developing our algorithms.
When we first develop a machine learning model, we must train it to learn about the data and extract data features. We do this using ‘training data’.
In our research, the training data was from a public dataset called the Cancer Imaging Archive and the Cancer Genomics Archive.
While this is a great starting point, one of the biggest concerns we have in machine learning model development is a problem called ‘overfitting’. This is when the model only performs well for the data it was trained with, and fails to generalise to new datasets. It is often a problem when the training dataset is small.
To avoid overfitting, we are using data from the National Genomic Research Library, much of which has been volunteered through the 100,000 Genomes Project.
By doing this, we have exposed the model to so many more images, helping to avoid overfitting, and ensuring that it learns robust and generalised patterns.
The scale and breadth of the National Genomic Research Library are enabling us to develop an accurate and effective model, with potential to be applied to patients beyond those who were part of the research.
Impact for patients
The main purpose of the work is to help ensure each patient gets the treatment best suited for them.
Specifically, we are investigating immunotherapy as a ‘neoadjuvant treatment’, meaning a treatment that is administered before the main therapy. We want to find out from the very beginning who is likely to respond well to this, so we can provide a personalised and targeted approach.
Our research here is a prospective study, because data has been collected over a long period of time. Our findings will help patients in the future, and anyone who has volunteered data has played a vital role in improving our tools for the next generation of patients.
What’s next for me?
I have been working on developing machine learning models using multi-modal data and fully managed machine learning service called Amazon SageMaker. This AWS multi-modal environment enables genomic data to be combined with other forms of medical data, providing a more holistic overview of a patient.
I would like to continue working on SageMaker and improving its machine learning skills.
I would also like to continue studying cancers, in particular glioblastoma, because I have learnt so much about it... although, our model has potential to be used on a range of cancers, so perhaps I will end up working on something different!
Beyond studying specific tumour characteristics, applying the model to different cancer types would be a very similar process.
My message to researchers
I would recommend everyone, not just early career researchers but all researchers, work with data from the National Genomic Research Library.
The dataset is constantly growing, and I’m confident this will continue. The scale of the data offers so many opportunities for new research, and as it grows, even more opportunities will arise.
And finally...
I would like to thank the Genomics England Multimodal Data team, specifically Dr Prabhu Arumugam and Dr Thomas Booth from Kings College London. Both were pivotal in helping me connect various sets of data for my research project, and were so patient and helpful along the way.
To find out more about opportunities at Genomics England, check out our early career researcher web page.
You can also apply to join the Genomics England academic research community if you are interested in accessing data from the National Genomic Research Library.