Research Network Communities
Join one of the Research Network communities to collaborate with fellow researchers, share skills and expertise, and learn about the latest developments and opportunities in the community.
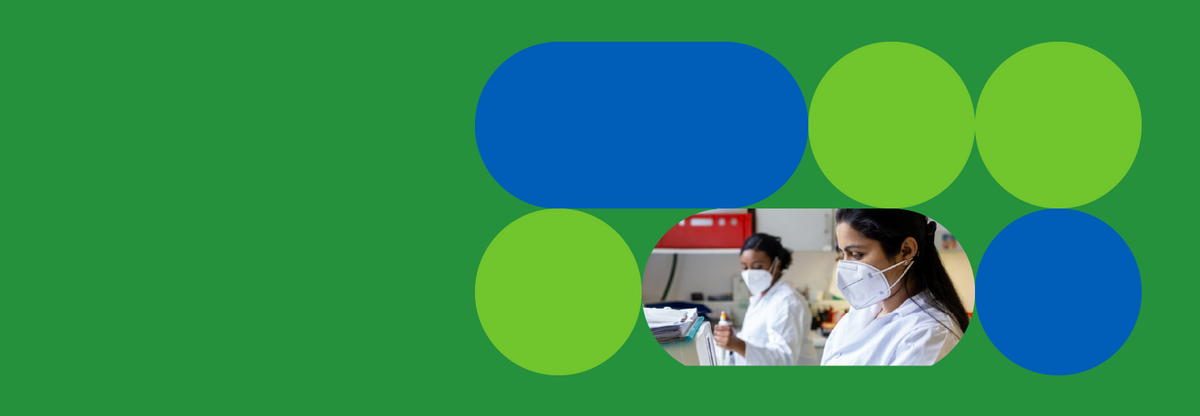
Bioinformatics and Machine Learning community
Chair: Professor Chris Yau
Co-Lead: Dr David Morris
Co-Lead: Dr Dominik Glodzik
Description:
Bioinformatics and machine learning are at the core of genomic research. They provide the algorithms and computational tools needed to investigate omics (e.g. genomics, proteomics, metabolomics) and clinical data, often at scale, to find meaningful associations and patterns. These include finding genotype–phenotype correlations, biomarker identification, gene function predictions, and pathogenicity scores, as well as mapping and annotating genomic features, such as enhancers, promoters, and splice sites. Research in this community will focus on the development, refinement, validation and application of bioinformatics approaches to interrogate data in the NGRL and maximise the ability for clinically relevant discovery. It will facilitate the open exchange and sharing of computational ideas and methods, including powerful machine learning approaches. It will also ensure best practices in clinical interpretation analysis, work with disease groups to provide access to world-leading statistical and computational data analysis expertise; and provide training and support a world-leading workforce in support of genomic-based healthcare.
Opportunities:
- Validation or expansion of machine learning and other computational algorithms/models on our real-world data dataset.
- Models and algorithms for variant prioritisation and inferring associations with phenotypes.
- Helping new researchers to understand what data exists, how it was collected and what computational analyses it can/cannot it be used for.
- Developing tailored on-boarding processes and “research-ready” datasets for machine learning researchers (training, process ready tools, etc).
- Creating cross-disciplinary training opportunities for researchers from other disciplines to enter computational genomics-based healthcare.
Genotype-Phenotype Association community
Chair: Professor Diana Baralle
Co-Lead: Dr Gavin Arno
Co-Lead: Dr Hywel Williams
Description:
Genotype-phenotype association studies seek to identify correlations between genomic variants and disease phenotypes at scale. Analysing the WGS data from the large patient cohorts available in the NGRL facilitates the discovery and characterization of rare genetic variants correlated with a trait or disease. These studies will improve our understanding of the aetiology of disease traits, identify potential new biological pathways underlying phenotypes, and contribute to clinical risk determinations.
The research of this community will seek to identify novel variant-trait associations and identify where such genotypic information can be leveraged for clinical application; such as the provision of polygenic risk scores and development of screening and treatment strategies. Particular attention will be given to the analysis of ancestries underrepresented in genomic research. This community will implement and improve tools for such analyses.
Opportunities:
- Validation or expansion of machine learning and other computational algorithms/models on our real-world data dataset.
- Models and algorithms for variant prioritisation and inferring associations with phenotypes.
- Predictions to identify the most and least likely variants to cause a phenotype.
- Statistical methods or bioinformatics pipelines for integrating data from multiple omics, e.g. for molecular characterisation of cancer types, or for prioritising variants and targets.
- Core statistical and computational methodologies for dealing with big data.
- Novel disease-gene associations
- Novel variant- disease associations.
- Leverage other omic- data and novel sequencing tools.
- Determine disease gene associations in diverse ancestries, improve ancestry data.
- Novel statistical and computational data analysis algorithms.
- Better understand the utility of Long Read sequencing technology DNA and RNA. Build on Long Read sequencing analysis tools and leverage this data for genomic discoveries.
Implementation and Data Enhancement community
Chair: Professor AJ McKnight
Co-Lead: Professor Claire Shovlin
Co-Lead: Dr James Buchanan
Description:
The NHS is the first national health care system to offer whole genome sequencing as part of routine care. This presents an enormous opportunity for the betterment of treatment and genomic research, but also creates significant practical challenges around the effective implementation and application of genomic medicine in the national healthcare ecosystem. Research in this community will explore the social, economic, legal, and ethical effectiveness of Genomic Medicine in the NHS, as well as work to improve data quality and availability.
Opportunities:
- Outputs that support and leverage data federation and the improvement of clinical and secondary health data in the NGRL.
- Quantitative and qualitative findings about the impact and efficacy of genomic medicine uptake and of the clinical and research uses of genomics.
- Patient reported outcomes.
- Measures of public perception and willingness to opt into genomic research.
Pan-Cancer and Molecular Oncology community
Chair: Professor Richard Houlston
Co-Lead: Professor Anna Schuh
Co-Lead: Professor Matt Lechner
Description:
While all cancers are molecularly distinct, reflecting their different cell types of origin and the myriad genomic alterations that promote oncogenesis, many tumours share similar genetic alterations that disrupt common biological processes. Charting this molecular landscape, including both commonalities and differences across tumour types, allows us to better understand the functional origins of cancers, define their molecular subtypes, identify clinically relevant biomarkers, and predict therapeutic response. The Pan-Cancer and Molecular Oncology community will carry out research across all tumour and germline samples present in the NGRL. It will use the data to identify and investigate driver mutations across tumours, to find functional alterations and impaired biological mechanisms common to all/many cancers, and to establish whether there are common mutational signatures that affect treatment efficacy and toxicity.
Opportunities:
- The detection of somatic genetic abnormalities underlying development of specific cancer types, including rare and noncoding driver mutations.
- Discovery of fundamental processes common to many or all cancer types (oncogenic processes).
- Identification of therapeutic targets for drug discovery or repurposing, or therapies effective in one cancer type that could be extended into others with a similar genomic profile.
- Identification of biomarkers to facilitate early detection and treatment.
- Exploration of new technologies and their ability to expand our understanding of cancer across the board.
Population Genomics community
Chair: Dr Aylwyn Scally
Co-Lead: Professor Jean-Baptiste Cazier
Co-Lead: Professor Patricia Munroe
Co-Lead: Dr Hilary Martin
Description:
The Population Genomics community includes researchers studying genomic variation in population-scale cohorts of individuals within the National Genomic Research Library (NGRL). It fosters research and collaboration using the data and resources in the NGRL and external datasets, including the development of methods, tools and new resources. This research aims to improve our understanding of human genetic variation and the processes that shape it, such as demography, ancestry, natural selection and genetic mutation. It also aims to elucidate the genomic contribution to disease and health in the population, and to inform diagnosis, treatment and the potential impact of interventions.
Opportunities:
The NGRL comprises population-wide genomic and phenotypic data, representing individuals from a wide diversity of ancestral, cultural and social backgrounds. In particular, this includes more than 10,000 whole-genome sequenced family trios as part of the rare disease cohort. Thus, it provides a valuable, and in many aspects unprecedented, opportunity for population genomic research.
Priority research areas include:
- Development of population genetic and computational methods for ancestral demographic inference.
- Understanding evolutionary genetic processes such as de novo mutation and structural variation.
- Applying machine learning and AI approaches to understanding genomic variation and function.
- Statistical modelling of genetic, environmental and other exposure-related factors in health and development.
- Methods for analysis and prediction combining a diversity of data types, including health records, biomarkers, functional genomics and metadata, in addition to genomic sequences.
Predisposition and Screening community
Chair: Professor Elijah Behr
Co-Lead: Professor Sanjay Sisodiya
Co-Lead: Professor Gianpiero Cavalleri
Description:
The human genome holds three billion base pairs of DNA, uniquely arranged to shape our individual characteristics and health. Alterations in the genetic code can directly cause or predispose individuals and families to rare diseases and cancer, as well as dictate the efficacy and toxicity of treatments, and influence co-morbidities. Genome sequencing provides a powerful tool to identify individuals at higher risk of developing disease and facilitates the accurate early diagnosis and personalized treatment that can radically improve patient life quality and prognosis. The Predisposition and Screening community will analyse genome data from families and individuals in the NGRL, including participants in the Generation Study and Diverse Data initiative, to identify de novo and inherited variants causing or predisposing to disease in diverse ancestries.
Opportunities:
- To identify de novo and inherited variants predisposing to disease or impact longitudinal disease progression, research for comorbidities and oligogenic and polygenic risk.
- To understand genetic epidemiology and assessment of effect of ancestry on predisposing variants and risk.
- To appreciate the differences in genomically mediated risk between general population and families affected by rare genetic diseases.
- To generate and refine polygenic risk scores for use in individuals and families as well as the general population.
- To promote development of population/public health screening strategies incorporating clinical and genomic data in novel AI-led models.
- To engage in cross-community collaborations and enhance interactions among Research Network members with complementary research interests.
Therapeutic Innovation and Trials community
Chair: Professor Danny Gale
Co-Lead: Professor Haiyan Zhou
Co-Lead: Professor Jenny Taylor
Description:
Genomics offers great opportunities for improved prediction, prevention, diagnosis and targeted treatment of disease. The translation of fundamental genomic discovery into patient benefit is a primary goal of the Research Network. This community will support research that propels genomic and clinical insight and discovery into therapeutic development and application.
Opportunities:
- Molecular markers to predict adverse reactions in trials or for therapeutics such as chemotherapy.
- Companion diagnostics and molecular targets for new therapies and more personalised or innovative treatments.
- Stratify genomics data to identify amenable targets for novel genetic therapies and precision medicine.
- Matching novel compounds with targets and drug repurposing of existing compounds, e.g. via shared molecular pathways.
- Design of and recruitment into clinical trials (trial design, patient identification and participation, reduction of attrition, therapeutic endpoints, RWE).
- Algorithms and tools to facilitate this research.
- Rapid clinical translation of novel genetic therapy and personalised medicine in patients with rare disease.
Variant Discovery and Clinical Interpretation community
Chair: Professor Sophie Hambleton
Co-Lead: Professor Claude Chelala
Co-Lead: Dr Alisdair McNeill
Description:
There are over 7000 recognized rare diseases which collectively impact 1 in every 17 people. The typical diagnostic journey spans eight years, and requires numerous medical assessments, resulting in a delay of optimal treatment. Genomic research is transforming our ability to diagnose rare disease and to optimize treatment for individual patients.
Research in this community will strive to provide molecular diagnoses for participants by identifying or validating variants that explain previously undiagnosed cases and feeding these discoveries back into clinical practice via the appropriate pathways. New tools and data will be leveraged to tackle challenges and improve of ability to rapidly and reliably identify disease variants.
Opportunities:
- New associations between a clinically defined phenotype and a genetic variant or variants, which explain previously undiagnosed cases.
- Identification of disease variants and disease mechanisms that inform clinical action and facilitate personalised treatment.
- Development of orthogonal tests for pathogenicity to ascertain the significance of novel variants, supporting screening, early diagnosis and early treatment of disease.
- Development of tools and resources to support diagnostic discovery.
Enablers:
- Sharing best practice and findings in the Diagnostic Discovery space, including between GEL, NHS and the Research Network.
- Identification of more participants with the genetic variants of interest.
- More granular and richer phenotypic descriptions of rare disease cases (e.g. through HES data/GP data, etc.), to uncover potential hidden disease-associated genomic variation.
- Tools to identify and predict the effect of splicing variants, epigenetic variants, non-coding variants, structural variants, etc.
Bioinformatics and Machine Learning community
Chair: Professor Chris Yau
Co-Lead: Dr David Morris
Co-Lead: Dr Dominik Glodzik
Description:
Bioinformatics and machine learning are at the core of genomic research. They provide the algorithms and computational tools needed to investigate omics (e.g. genomics, proteomics, metabolomics) and clinical data, often at scale, to find meaningful associations and patterns. These include finding genotype–phenotype correlations, biomarker identification, gene function predictions, and pathogenicity scores, as well as mapping and annotating genomic features, such as enhancers, promoters, and splice sites. Research in this community will focus on the development, refinement, validation and application of bioinformatics approaches to interrogate data in the NGRL and maximise the ability for clinically relevant discovery. It will facilitate the open exchange and sharing of computational ideas and methods, including powerful machine learning approaches. It will also ensure best practices in clinical interpretation analysis, work with disease groups to provide access to world-leading statistical and computational data analysis expertise; and provide training and support a world-leading workforce in support of genomic-based healthcare.
Opportunities:
- Validation or expansion of machine learning and other computational algorithms/models on our real-world data dataset.
- Models and algorithms for variant prioritisation and inferring associations with phenotypes.
- Helping new researchers to understand what data exists, how it was collected and what computational analyses it can/cannot it be used for.
- Developing tailored on-boarding processes and “research-ready” datasets for machine learning researchers (training, process ready tools, etc).
- Creating cross-disciplinary training opportunities for researchers from other disciplines to enter computational genomics-based healthcare.
Genotype-Phenotype Association community
Chair: Professor Diana Baralle
Co-Lead: Dr Gavin Arno
Co-Lead: Dr Hywel Williams
Description:
Genotype-phenotype association studies seek to identify correlations between genomic variants and disease phenotypes at scale. Analysing the WGS data from the large patient cohorts available in the NGRL facilitates the discovery and characterization of rare genetic variants correlated with a trait or disease. These studies will improve our understanding of the aetiology of disease traits, identify potential new biological pathways underlying phenotypes, and contribute to clinical risk determinations.
The research of this community will seek to identify novel variant-trait associations and identify where such genotypic information can be leveraged for clinical application; such as the provision of polygenic risk scores and development of screening and treatment strategies. Particular attention will be given to the analysis of ancestries underrepresented in genomic research. This community will implement and improve tools for such analyses.
Opportunities:
- Validation or expansion of machine learning and other computational algorithms/models on our real-world data dataset.
- Models and algorithms for variant prioritisation and inferring associations with phenotypes.
- Predictions to identify the most and least likely variants to cause a phenotype.
- Statistical methods or bioinformatics pipelines for integrating data from multiple omics, e.g. for molecular characterisation of cancer types, or for prioritising variants and targets.
- Core statistical and computational methodologies for dealing with big data.
- Novel disease-gene associations
- Novel variant- disease associations.
- Leverage other omic- data and novel sequencing tools.
- Determine disease gene associations in diverse ancestries, improve ancestry data.
- Novel statistical and computational data analysis algorithms.
- Better understand the utility of Long Read sequencing technology DNA and RNA. Build on Long Read sequencing analysis tools and leverage this data for genomic discoveries.
Implementation and Data Enhancement community
Chair: Professor AJ McKnight
Co-Lead: Professor Claire Shovlin
Co-Lead: Dr James Buchanan
Description:
The NHS is the first national health care system to offer whole genome sequencing as part of routine care. This presents an enormous opportunity for the betterment of treatment and genomic research, but also creates significant practical challenges around the effective implementation and application of genomic medicine in the national healthcare ecosystem. Research in this community will explore the social, economic, legal, and ethical effectiveness of Genomic Medicine in the NHS, as well as work to improve data quality and availability.
Opportunities:
- Outputs that support and leverage data federation and the improvement of clinical and secondary health data in the NGRL.
- Quantitative and qualitative findings about the impact and efficacy of genomic medicine uptake and of the clinical and research uses of genomics.
- Patient reported outcomes.
- Measures of public perception and willingness to opt into genomic research.
Pan-Cancer and Molecular Oncology community
Chair: Professor Richard Houlston
Co-Lead: Professor Anna Schuh
Co-Lead: Professor Matt Lechner
Description:
While all cancers are molecularly distinct, reflecting their different cell types of origin and the myriad genomic alterations that promote oncogenesis, many tumours share similar genetic alterations that disrupt common biological processes. Charting this molecular landscape, including both commonalities and differences across tumour types, allows us to better understand the functional origins of cancers, define their molecular subtypes, identify clinically relevant biomarkers, and predict therapeutic response. The Pan-Cancer and Molecular Oncology community will carry out research across all tumour and germline samples present in the NGRL. It will use the data to identify and investigate driver mutations across tumours, to find functional alterations and impaired biological mechanisms common to all/many cancers, and to establish whether there are common mutational signatures that affect treatment efficacy and toxicity.
Opportunities:
- The detection of somatic genetic abnormalities underlying development of specific cancer types, including rare and noncoding driver mutations.
- Discovery of fundamental processes common to many or all cancer types (oncogenic processes).
- Identification of therapeutic targets for drug discovery or repurposing, or therapies effective in one cancer type that could be extended into others with a similar genomic profile.
- Identification of biomarkers to facilitate early detection and treatment.
- Exploration of new technologies and their ability to expand our understanding of cancer across the board.
Population Genomics community
Chair: Dr Aylwyn Scally
Co-Lead: Professor Jean-Baptiste Cazier
Co-Lead: Professor Patricia Munroe
Co-Lead: Dr Hilary Martin
Description:
The Population Genomics community includes researchers studying genomic variation in population-scale cohorts of individuals within the National Genomic Research Library (NGRL). It fosters research and collaboration using the data and resources in the NGRL and external datasets, including the development of methods, tools and new resources. This research aims to improve our understanding of human genetic variation and the processes that shape it, such as demography, ancestry, natural selection and genetic mutation. It also aims to elucidate the genomic contribution to disease and health in the population, and to inform diagnosis, treatment and the potential impact of interventions.
Opportunities:
The NGRL comprises population-wide genomic and phenotypic data, representing individuals from a wide diversity of ancestral, cultural and social backgrounds. In particular, this includes more than 10,000 whole-genome sequenced family trios as part of the rare disease cohort. Thus, it provides a valuable, and in many aspects unprecedented, opportunity for population genomic research.
Priority research areas include:
- Development of population genetic and computational methods for ancestral demographic inference.
- Understanding evolutionary genetic processes such as de novo mutation and structural variation.
- Applying machine learning and AI approaches to understanding genomic variation and function.
- Statistical modelling of genetic, environmental and other exposure-related factors in health and development.
- Methods for analysis and prediction combining a diversity of data types, including health records, biomarkers, functional genomics and metadata, in addition to genomic sequences.
Predisposition and Screening community
Chair: Professor Elijah Behr
Co-Lead: Professor Sanjay Sisodiya
Co-Lead: Professor Gianpiero Cavalleri
Description:
The human genome holds three billion base pairs of DNA, uniquely arranged to shape our individual characteristics and health. Alterations in the genetic code can directly cause or predispose individuals and families to rare diseases and cancer, as well as dictate the efficacy and toxicity of treatments, and influence co-morbidities. Genome sequencing provides a powerful tool to identify individuals at higher risk of developing disease and facilitates the accurate early diagnosis and personalized treatment that can radically improve patient life quality and prognosis. The Predisposition and Screening community will analyse genome data from families and individuals in the NGRL, including participants in the Generation Study and Diverse Data initiative, to identify de novo and inherited variants causing or predisposing to disease in diverse ancestries.
Opportunities:
- To identify de novo and inherited variants predisposing to disease or impact longitudinal disease progression, research for comorbidities and oligogenic and polygenic risk.
- To understand genetic epidemiology and assessment of effect of ancestry on predisposing variants and risk.
- To appreciate the differences in genomically mediated risk between general population and families affected by rare genetic diseases.
- To generate and refine polygenic risk scores for use in individuals and families as well as the general population.
- To promote development of population/public health screening strategies incorporating clinical and genomic data in novel AI-led models.
- To engage in cross-community collaborations and enhance interactions among Research Network members with complementary research interests.
Therapeutic Innovation and Trials community
Chair: Professor Danny Gale
Co-Lead: Professor Haiyan Zhou
Co-Lead: Professor Jenny Taylor
Description:
Genomics offers great opportunities for improved prediction, prevention, diagnosis and targeted treatment of disease. The translation of fundamental genomic discovery into patient benefit is a primary goal of the Research Network. This community will support research that propels genomic and clinical insight and discovery into therapeutic development and application.
Opportunities:
- Molecular markers to predict adverse reactions in trials or for therapeutics such as chemotherapy.
- Companion diagnostics and molecular targets for new therapies and more personalised or innovative treatments.
- Stratify genomics data to identify amenable targets for novel genetic therapies and precision medicine.
- Matching novel compounds with targets and drug repurposing of existing compounds, e.g. via shared molecular pathways.
- Design of and recruitment into clinical trials (trial design, patient identification and participation, reduction of attrition, therapeutic endpoints, RWE).
- Algorithms and tools to facilitate this research.
- Rapid clinical translation of novel genetic therapy and personalised medicine in patients with rare disease.
Variant Discovery and Clinical Interpretation community
Chair: Professor Sophie Hambleton
Co-Lead: Professor Claude Chelala
Co-Lead: Dr Alisdair McNeill
Description:
There are over 7000 recognized rare diseases which collectively impact 1 in every 17 people. The typical diagnostic journey spans eight years, and requires numerous medical assessments, resulting in a delay of optimal treatment. Genomic research is transforming our ability to diagnose rare disease and to optimize treatment for individual patients.
Research in this community will strive to provide molecular diagnoses for participants by identifying or validating variants that explain previously undiagnosed cases and feeding these discoveries back into clinical practice via the appropriate pathways. New tools and data will be leveraged to tackle challenges and improve of ability to rapidly and reliably identify disease variants.
Opportunities:
- New associations between a clinically defined phenotype and a genetic variant or variants, which explain previously undiagnosed cases.
- Identification of disease variants and disease mechanisms that inform clinical action and facilitate personalised treatment.
- Development of orthogonal tests for pathogenicity to ascertain the significance of novel variants, supporting screening, early diagnosis and early treatment of disease.
- Development of tools and resources to support diagnostic discovery.
Enablers:
- Sharing best practice and findings in the Diagnostic Discovery space, including between GEL, NHS and the Research Network.
- Identification of more participants with the genetic variants of interest.
- More granular and richer phenotypic descriptions of rare disease cases (e.g. through HES data/GP data, etc.), to uncover potential hidden disease-associated genomic variation.
- Tools to identify and predict the effect of splicing variants, epigenetic variants, non-coding variants, structural variants, etc.